Merge with Categorical as join key + how='inner' returns wrong output #21443
Labels
Duplicate Report
Duplicate issue or pull request
Reshaping
Concat, Merge/Join, Stack/Unstack, Explode
Code Sample, a copy-pastable example if possible
Problem description
According to Pandas 0.23.0 doc :
for the keyword "how", the especially "inner" config (bolded part):
So the merge is supposed to preserve the order of the left keys.
However, if Categorical Data is used as the key to merge, the order is not preserved.
The output dataframe has been sorted as per the join key, and the index has been reseted.
Which obviously provoke some unwanted results.
Note that how='left' does provide the correct results (regarding key ordering).
Expected Output
Expected Output :
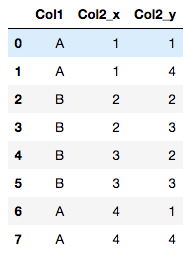
Actual Output :
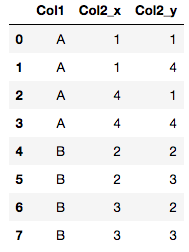
Output of
pd.show_versions()
INSTALLED VERSIONS
commit: None
python: 2.7.14.final.0
python-bits: 64
OS: Linux
OS-release: 4.13.0-38-generic
machine: x86_64
processor: x86_64
byteorder: little
LC_ALL: None
LANG: en_US.UTF-8
LOCALE: None.None
pandas: 0.23.0
pytest: None
pip: 9.0.1
setuptools: 36.5.0.post20170921
Cython: None
numpy: 1.14.3
scipy: 1.1.0
pyarrow: None
xarray: None
IPython: 5.4.1
sphinx: None
patsy: None
dateutil: 2.6.1
pytz: 2018.4
blosc: None
bottleneck: None
tables: None
numexpr: None
feather: None
matplotlib: None
openpyxl: None
xlrd: None
xlwt: None
xlsxwriter: None
lxml: None
bs4: None
html5lib: 0.9999999
sqlalchemy: None
pymysql: None
psycopg2: None
jinja2: 2.9.6
s3fs: None
fastparquet: None
pandas_gbq: None
pandas_datareader: None
@chrish42
The text was updated successfully, but these errors were encountered: